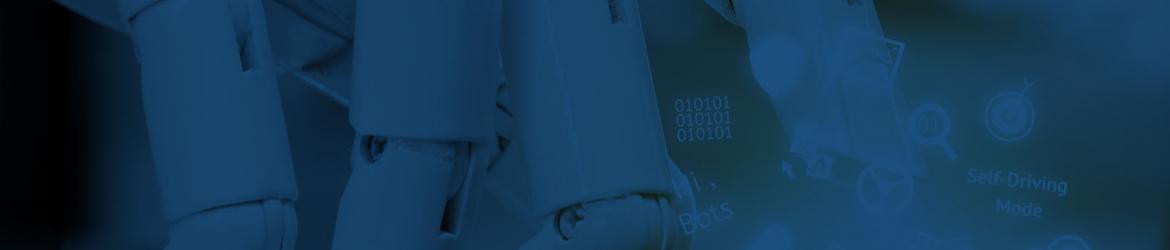
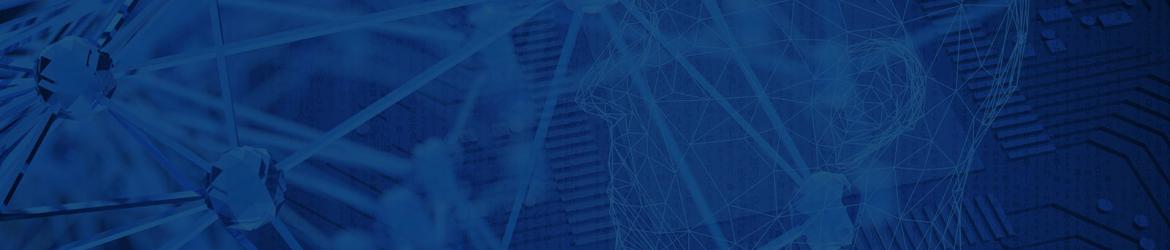
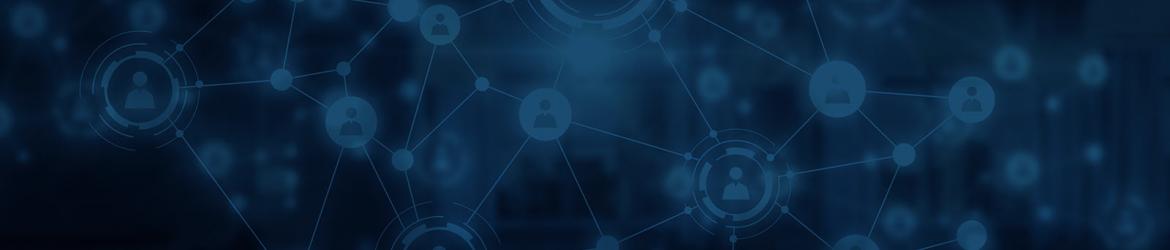
This dataset includes 1,000 meshes for each of 100 categories of fruits and vegetables. A sub-sample is currently available, and the full dataset will be released in the coming weeks.
Set of deteriorated versions of the publicly available non-commercial IMDB database, comprising different amounts of duplicates.
The datasets were extracted from PostgreSQL databases including relations titles, name_basics, title_episode, title_ratings, and title_principals from https://datasets.imdbws.com/ IMDB database version downloaded on April 7th, 2024. these databases were deteriorated on purpose to experiment the Red2Hunt method that generates a redundant-free database from any relational operational database comprising surrogate keys and duplicates.
A set of computer generated cave system and tunnel system
This dataset has been generated from 3 constructions models, transferred from Autodesk Revit to NVIDIA Isaac Sim. It contains 8751 samples of RGB images associated with the semantic segmentation masks and label files for 13 classes (rectangular_sheath, circular_sheath, pipe, air_vent, fan_coil, stair, wall, floor, pipe_accessory, framework, radiant_panel, climate_engineering_equipment, ceiling, handrail, roof, cable_tray, pole).
This dataset contains CSV and SQLite files with data about projects backends extracted from Metadata about every file uploaded to PyPI :
Source code for the data extraction.
(1) There are several pyproject.toml files for some projects (e.g poetry), often in test folders
(2) The test is quite basic, but there are few projects that have several pyproject.toml file matching this test
After the publication of the first charts, I wanted to know how many projects had no source package, how many projects had no pyproject.toml to complete the first statistics.
This dataset contains CSV and SQLite files extracted from the same source (parquet files from "Metadata about every file uploaded to PyPI"):
These files weight 1.1 and 1.3 Go respectively.
Source code for the second data extraction.
This dataset holds
This dataset originates from the AMETHYST project. It comprises a collection of PDFs and images that undergo Machine Learning and NLP processing to extract tables containing information about Epoxy/Amine (EA) compounds and their properties.
FruitBin contains more than 1M images and 40M instance-level 6D pose annotations over both symmetric and asymmetric fruits with or without texture. Rich annotations and metadata (including 6D pose, segmentation mask, point cloud, 2D and 3D bounding boxes, occlusion rate) allow the tuning of the proposed dataset for benchmarking the robustness of object instance segmentation and 6D pose estimation models (with respect to variations in terms of lighting, texture, occlusion, camera pose and scenes). We further propose three scenarios presenting significant challenges of 6D pose estimation models: new scene generalization; new camera viewpoint generalization; and occlusion robustness. We show the results of these three scenarios for two 6D pose estimation baselines making use of RGB or RGBD images. To the best of our knowledge, FruitBin is the first dataset for the challenging task of fruit bin picking and the biggest large-scale dataset for 6D pose estimation with the most comprehensive challenges, tunable over scenes, camera poses and occlusions.
License : CC BY-NC-SA
Estimating fluid dynamics is classically done through the simulation and integration of numerical models solving the Navier-Stokes equations, which is computationally complex and time-consuming even on high-end hardware. This is a notoriously hard problem to solve, which has recently been addressed with machine learning, in particular graph neural networks (GNN) and variants trained and evaluated on datasets of static objects in static scenes with fixed geometry. We attempt to go beyond existing work in complexity and introduce a new model, method and benchmark. We propose EAGLE, a large-scale dataset of ∼1.1 million 2D meshes resulting from simulations of unsteady fluid dynamics caused by a moving flow source interacting with nonlinear scene structure, comprised of 600 different scenes of three different types. To perform future forecasting of pressure and velocity on the challenging EAGLE dataset, we introduce a new mesh transformer. It leverages node clustering, graph pooling and global attention to learn long-range dependencies between spatially distant data points without needing a large number of iterations, as existing GNN methods do. We show that our transformer outperforms state-of-the-art performance on, both, existing synthetic and real datasets and on EAGLE. Finally, we highlight that our approach learns to attend to airflow, integrating complex information in a single iteration.
A collection of urban data graphs in RDF/OWL formats derived from CityGML Grand Lyon Open data